Open-source intelligence (OSINT) has become an essential part of modern investigations, threat analysis, and decision-making. By leveraging publicly available information from the surface web, social media, forums, and more obscure corners of the internet, OSINT practitioners can uncover insights without the need for intrusive or covert methods. But as with any form of intelligence gathering, the process is far from objective.
Bias — whether introduced by the analyst, the tools used, or the sources themselves — can significantly distort findings. In an age where data is vast, varied, and often unverified, understanding and mitigating bias is not just good practice, it’s a necessity.
In this blog post, we’ll explore the different types of bias that can affect OSINT, from unconscious assumptions to platform-driven distortions. We’ll also look at the real-world consequences of unchecked bias and offer practical steps to help analysts and organisations reduce its impact. Because when it comes to intelligence, clarity and objectivity are key — and bias is the silent threat that clouds both.
What Is Bias in OSINT?
Bias, in the context of OSINT, refers to any distortion or influence that affects how information is collected, interpreted, or presented. It can arise from a wide range of sources — the tools we use, the platforms we search, the assumptions we bring with us, and even the way we frame our intelligence requirements.
Importantly, bias isn’t always intentional. Much of it operates at a subconscious level, shaped by cultural norms, past experiences, professional habits, or institutional practices. And in OSINT — where we often deal with vast, unstructured, and fast-moving data — even small biases can significantly skew the outcome of an investigation.
Bias can enter the OSINT process at every stage. It may influence the types of sources we prioritise, the way we interpret ambiguous content, or the confidence we place in particular findings. Analysts may unconsciously favour information that supports a working theory, or dismiss data that doesn’t align with an expected narrative. Meanwhile, digital tools and search algorithms can subtly reinforce these patterns, feeding analysts what they’re likely to click on — not necessarily what’s most accurate or relevant.
Recognising the presence of bias is the first step in mitigating its effects. In the sections that follow, we’ll explore some of the most common types of bias in OSINT work, and how they can impact the quality and reliability of our intelligence.
Types of Bias Common in OSINT
Bias in OSINT can creep in at any stage of the intelligence lifecycle — from the moment an analyst frames a question, to the sources they choose, the tools they rely on, and how they interpret the information gathered. These biases, often unconscious, can impact the reliability, relevance, and objectivity of an intelligence product. Below are the most prevalent types of bias that OSINT practitioners should be aware of, alongside examples and mitigation tips.
Selection Bias
Selection bias arises when the information an analyst collects is not representative of the broader landscape, often because certain types of sources or platforms are favoured over others. This can be due to habit, language familiarity, ease of access, or time constraints.
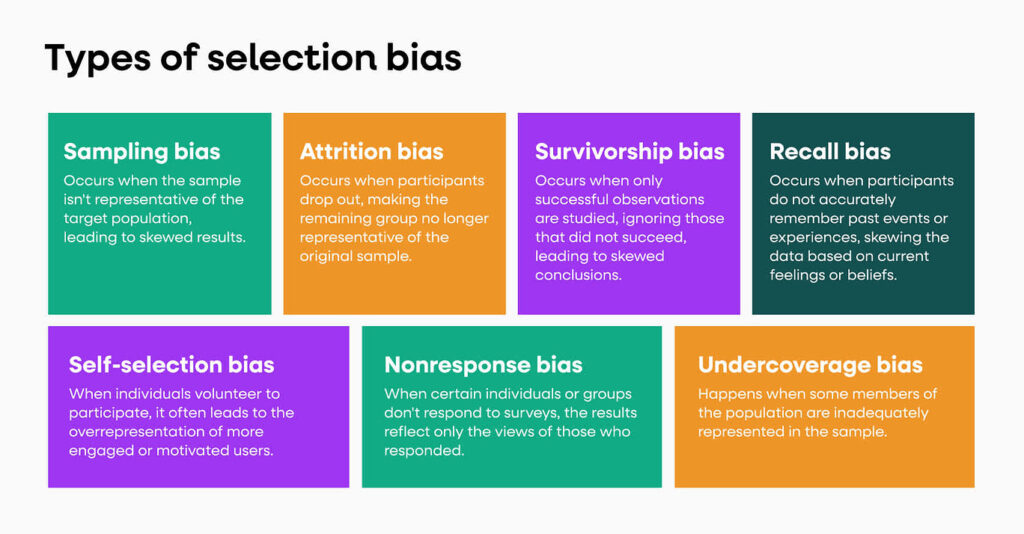
Example:
An analyst researching political disinformation may rely heavily on Twitter data, missing coordinated narratives being pushed on Telegram or region-specific platforms like VK or Weibo.
Why it matters:
If the selected sources don’t reflect the full spectrum of available information, the resulting intelligence may be incomplete, misleading, or skewed towards a particular narrative or demographic.
How to reduce it:
- Use a diverse set of platforms and media types (forums, blogs, videos, alt-tech sites).
- Include regional and language-specific sources wherever possible.
- Revisit and regularly reassess your go-to sources to prevent over-reliance.
Confirmation Bias
Confirmation bias is the tendency to look for or interpret information in a way that supports an existing hypothesis or belief, while disregarding evidence that contradicts it. This is especially common when an analyst is under pressure to produce a “smoking gun” or validate a stakeholder’s expectations.
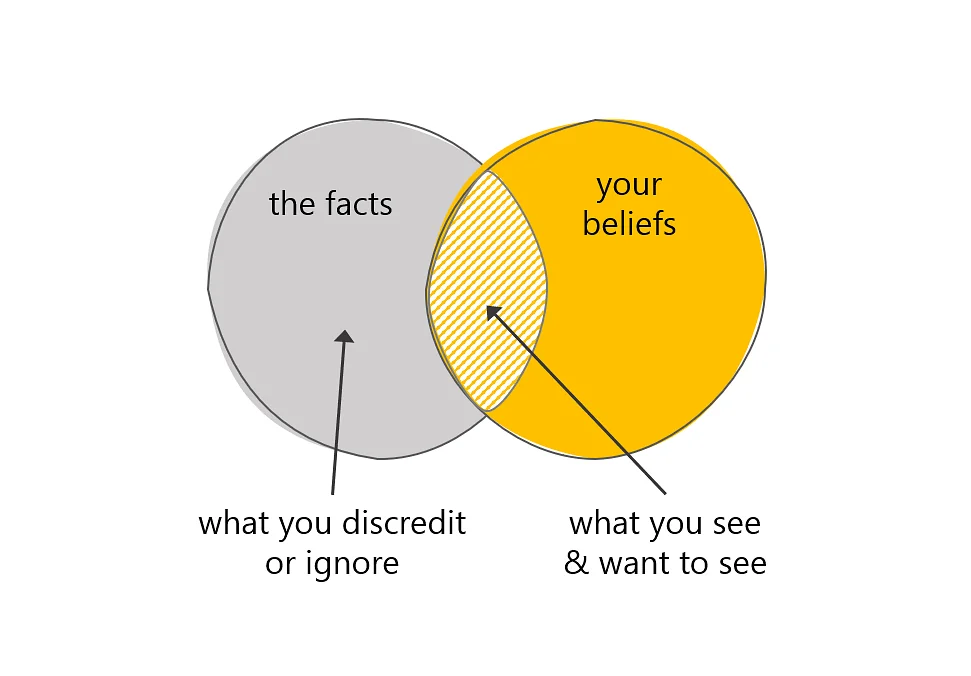
Example:
While investigating a suspected nation-state actor, an analyst focuses exclusively on TTPs (Tactics, Techniques, and Procedures) associated with that actor, ignoring signs that the activity could point to a different group or a false flag operation.
Why it matters:
Confirmation bias can lead to poor attribution, misinformed decisions, or ineffective mitigation strategies. It also limits an analyst’s ability to explore alternative hypotheses.
How to reduce it:
- Apply structured analytic techniques such as the Analysis of Competing Hypotheses (ACH).
- Collaborate with other analysts to test assumptions and encourage critical challenge.
- Document reasoning and acknowledge uncertainty in assessments.
Language and Cultural Bias
Language barriers and cultural unfamiliarity can affect how information is gathered and interpreted. Analysts working in a second language — or relying on machine translation — may misread tone, sarcasm, or idiomatic expressions. Cultural norms can also impact how certain behaviours are perceived.
Example:
An English-speaking analyst may misinterpret the tone of Arabic-language social media posts due to literal translation, mistaking satire or frustration for calls to violence.
Why it matters:
Poor interpretation can lead to false positives, mischaracterisation of intent, or overlooking local context. This is particularly critical in geopolitical, extremist, or criminal investigations.
How to reduce it:
- Use native speakers or trusted translation partners when possible.
- Consult regional experts for cultural insight.
- Avoid making assumptions based solely on automated translations or surface-level interpretations.
Tool and Platform Bias
The tools we use to collect and analyse data are not neutral. Search engines, social media platforms, and scraping tools all apply filters, ranking algorithms, and personalisation — often without the user’s awareness. This can prioritise certain types of content and bury others, skewing the analyst’s perception of what is prevalent or important.
Example:
Google search results vary depending on location, search history, and user profile. An analyst may believe a narrative is trending globally when in fact it’s only prominent in their localised feed.
Why it matters:
Platform bias can lead to a false sense of consensus or popularity. It also risks amplifying certain voices while suppressing dissenting ones.
How to reduce it:
- Use multiple search engines and anonymised browsers (e.g. Tor or VPNs).
- Test queries in incognito/private browsing modes.
- Be aware of default settings in commercial tools — understand what’s being excluded or prioritised.
Data Availability Bias
Data availability bias refers to the over-reliance on information that is easiest to find, most recent, or most abundant. Analysts may gravitate towards high-volume data sources (like Reddit or Twitter) because they are continuously updated and easy to search — at the expense of smaller, less visible sources that may be more valuable.
Example:
An OSINT report on cybercriminal activity may cite dozens of tweets and blog posts but fail to include key discussions taking place in closed forums or encrypted messaging groups.
Why it matters:
The quantity of available data doesn’t always equate to quality or relevance. Prioritising what’s visible over what’s essential can distort the intelligence picture and give a false sense of completeness.
How to reduce it:
- Establish clear intelligence requirements before collection begins.
- Allocate time to seek out hard-to-find or niche sources.
- Treat gaps in data as a signal — not just an absence.
Together, these biases form a web of influence that can compromise even the most well-intentioned investigations.
Real-World Consequences of Bias in OSINT
Bias in OSINT isn’t just a theoretical concern — it has real-world implications. When unchecked, bias can lead to flawed assessments, damaged reputations, operational missteps, and even legal or ethical breaches. Whether you’re conducting corporate investigations, monitoring geopolitical events, or assessing cyber threats, the integrity of your findings depends on how rigorously you confront bias throughout the process.
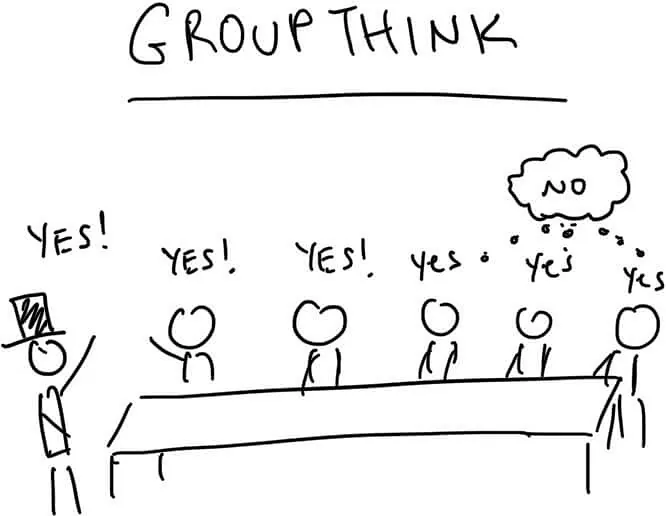
Here are some key consequences of biased OSINT!
Flawed Decision-Making
Biased intelligence can feed directly into poor decisions, especially in fast-moving environments where leadership relies heavily on OSINT to shape strategy or response.
Example:
A security team monitoring social unrest misinterprets online sentiment due to over-reliance on English-language Twitter data. As a result, they misjudge the timing and location of protest activity, leading to inadequate resource allocation and reputational damage for the organisation.
Impact:
Misinformed decisions can result in financial losses, safety risks, or missed opportunities to intervene early in an emerging threat.
Inaccurate Attribution and Threat Profiling
In cyber threat intelligence, OSINT is often used to support attribution — linking incidents to actors or groups. Bias in source selection or interpretation can lead to false conclusions about who is behind an attack or what their motives might be.
Example:
An analyst attributes a phishing campaign to a well-known ransomware gang based on superficial similarities to a past incident, without exploring the possibility of copycat tactics. Later evidence reveals the activity was the work of a different actor altogether.
Impact:
Faulty attribution may lead to targeting the wrong group, damaging diplomatic relationships, or overlooking the true threat actor.
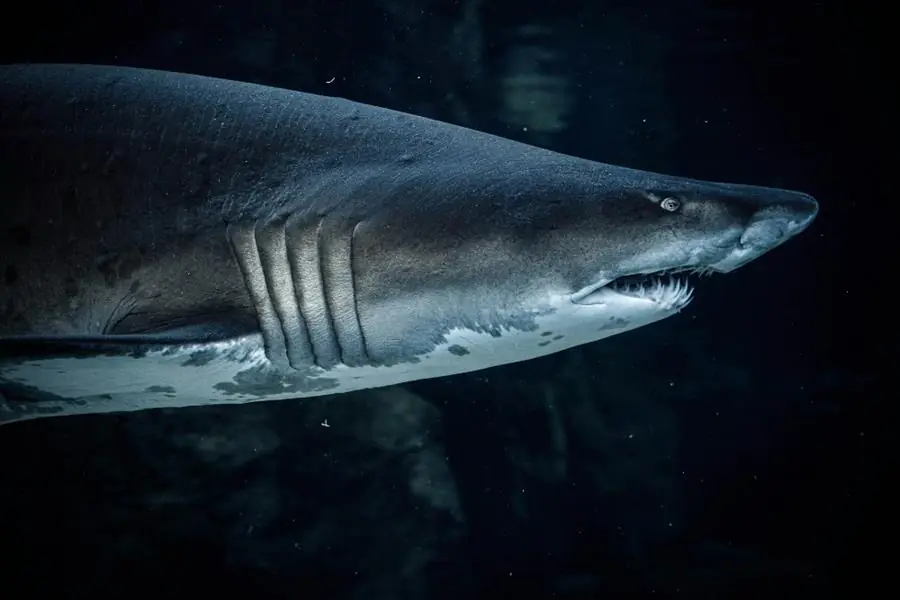
Overlooking Emerging Threats
Bias towards mainstream or high-visibility platforms can cause analysts to miss activity in fringe spaces where new narratives or tactics often emerge first.
Example:
While monitoring disinformation around an election, analysts focus on Facebook and YouTube but fail to detect early mobilisation efforts on fringe platforms like 4chan or niche messaging channels.
Impact:
Failure to detect early-stage planning or sentiment shifts can delay mitigation efforts and allow threats to escalate unchecked.
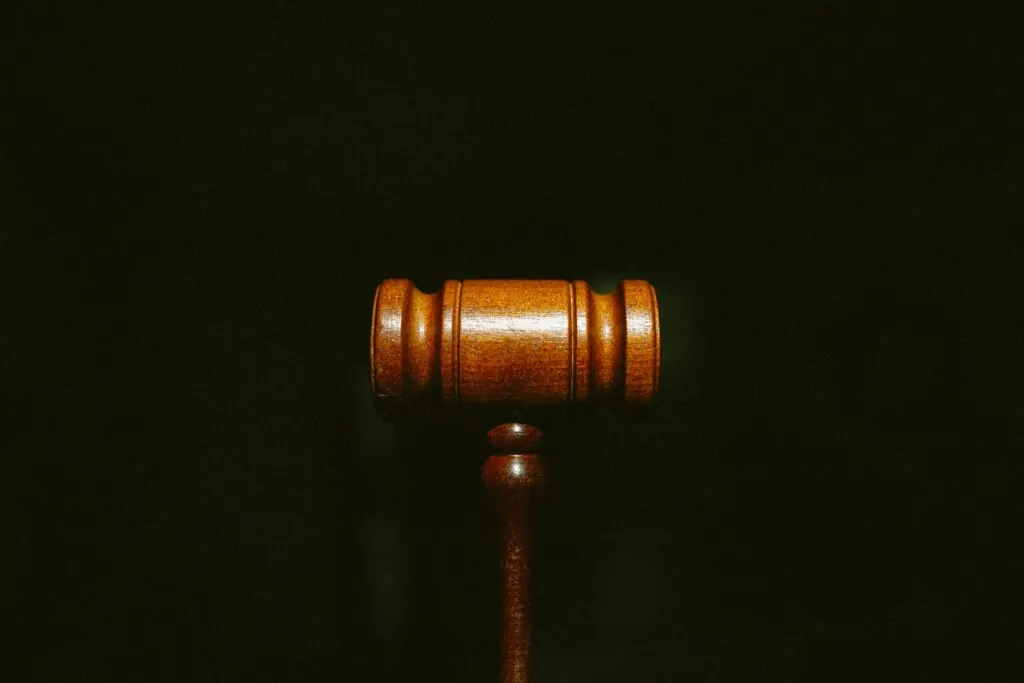
Reputational and Legal Risks
If an organisation bases public statements or internal actions on flawed OSINT, it could face reputational fallout — or worse, legal consequences.
Example:
A company issues a threat advisory naming a suspected actor based on an OSINT report later revealed to be based on misinterpreted data. The accused actor contests the findings publicly, leading to reputational damage and potential liability.
Impact:
Poorly substantiated claims can erode trust in your organisation’s intelligence capabilities and create significant legal exposure.
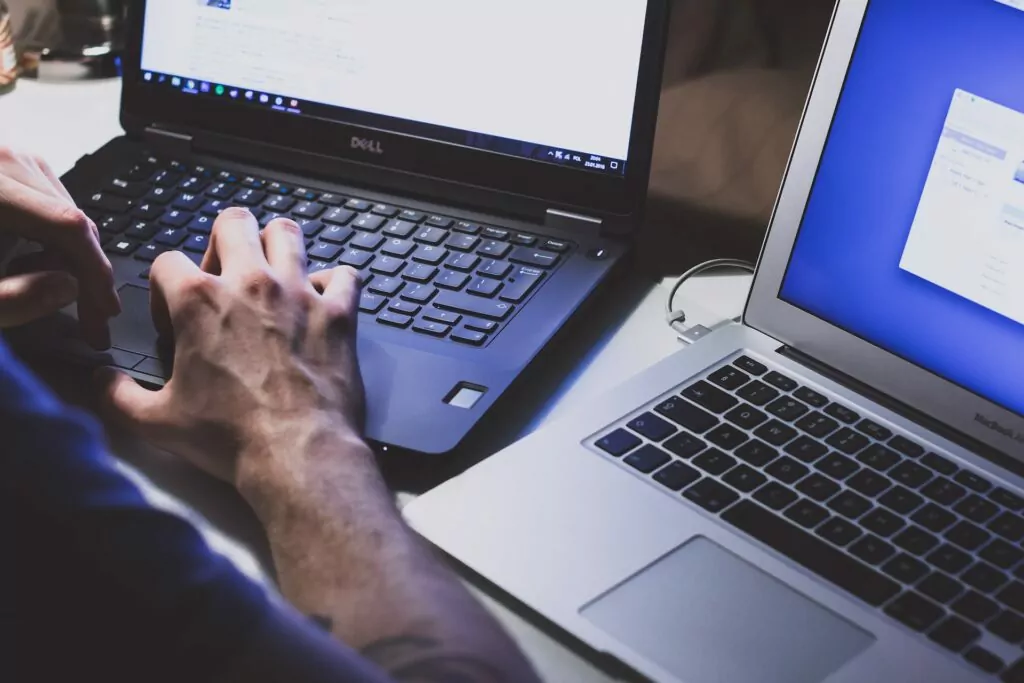
Analyst Burnout and Operational Inefficiency
Constantly chasing data that confirms a pre-existing view can lead to tunnel vision and missed insight. It also increases cognitive load, as analysts struggle to reconcile contradictory findings with an inflexible narrative.
Example:
An intelligence team spends weeks reinforcing an incorrect assumption because early findings were never challenged. Late-stage doubts lead to rework and missed deadlines.
Impact:
Bias drains time, undermines analyst confidence, and reduces the overall efficiency of the OSINT process.
By understanding and acknowledging these consequences, OSINT professionals can treat bias not just as a theoretical flaw but as a practical risk — one that can and should be actively mitigated. In the next section, we’ll explore how to do exactly that: recognising bias in your own process, and adopting safeguards to reduce its impact.
How to Identify and Mitigate Bias in OSINT Investigations
Tackling bias in OSINT isn’t about eliminating it entirely — that’s virtually impossible. Instead, the goal is to recognise where bias may creep in, actively question your assumptions, and build safeguards into your processes to keep your intelligence as accurate, balanced, and reliable as possible. Below are key strategies for identifying and mitigating bias throughout the OSINT lifecycle.
Develop Self-Awareness and Encourage Critical Thinking
Awareness is the first step. Bias is often unconscious, so analysts must learn to reflect on their own thought processes and remain open to challenge.
Tips:
- Ask yourself: “What assumptions am I making here?”
- Encourage peer review within your team — a second set of eyes can catch blind spots you might miss.
- Maintain a mindset of curiosity over certainty. Avoid becoming too attached to an early hypothesis.
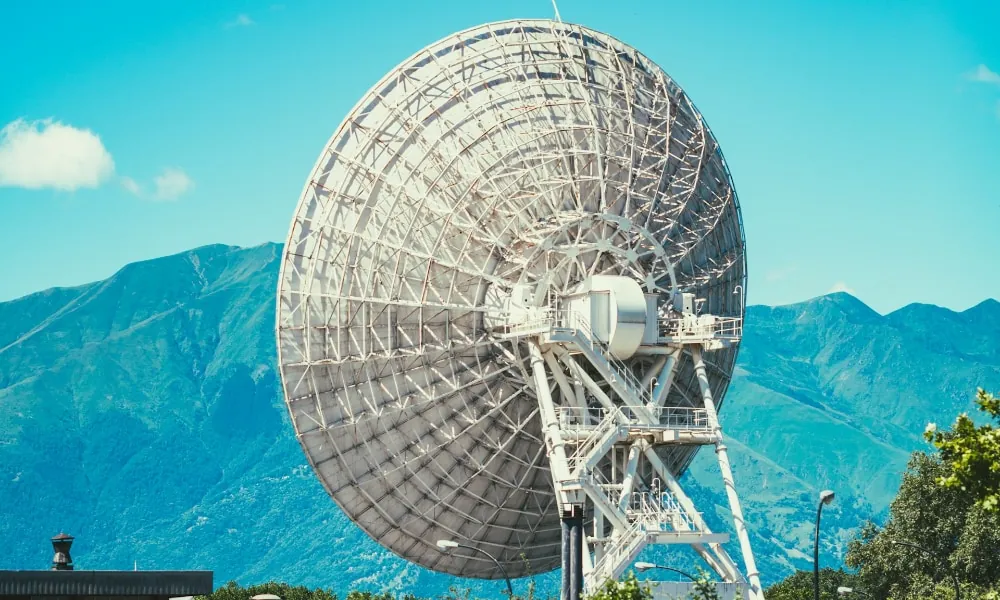
Use Structured Analytic Techniques (SATs)
Structured Analytic Techniques are proven tools to help analysts explore alternative explanations, test assumptions, and reduce cognitive traps.
Recommended techniques:
- Analysis of Competing Hypotheses (ACH): List all possible explanations and evaluate evidence for and against each.
- Red Teaming: Have a colleague deliberately challenge your assumptions and present counter-arguments.
- Devil’s Advocacy: Take an opposing viewpoint to test the strength of your conclusions.
These methods are particularly valuable in high-stakes or high-uncertainty investigations where bias may have the greatest impact.
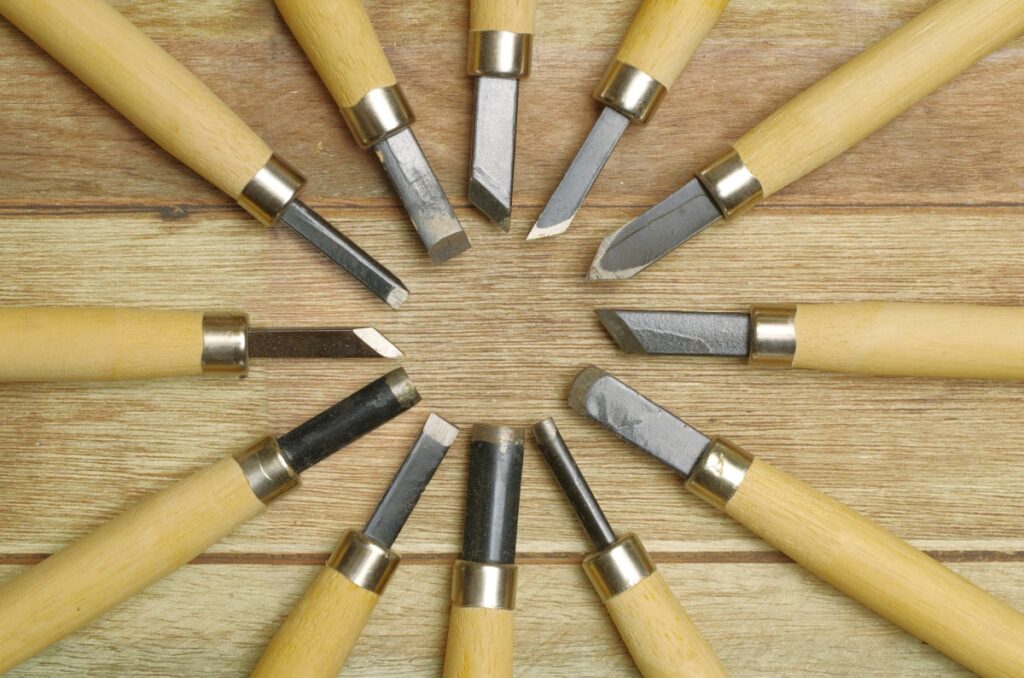
Diversify Sources and Tools
One of the most effective ways to reduce selection and tool bias is to cast a wide net. Avoid relying on a narrow set of familiar platforms or sources.
Tips:
- Include mainstream, alternative, and fringe platforms in your data collection.
- Use both commercial and open-source OSINT tools — each may present data differently.
- Search in multiple languages where possible, or use translated queries to gain a broader view.
Regularly audit your sources and collection methods to ensure they remain appropriate for the task.
Separate Collection from Analysis
Where feasible, keep data collection and analysis distinct. This can help prevent your search strategy from being shaped by what you hope to find.
Tips:
- Assign data gathering to one team member and analysis to another, if resources allow.
- Use neutral search terms during collection to avoid biasing the dataset.
- Create a clear intelligence requirement or question to guide your scope objectively.
This separation adds discipline to your workflow and supports a more neutral intelligence product.
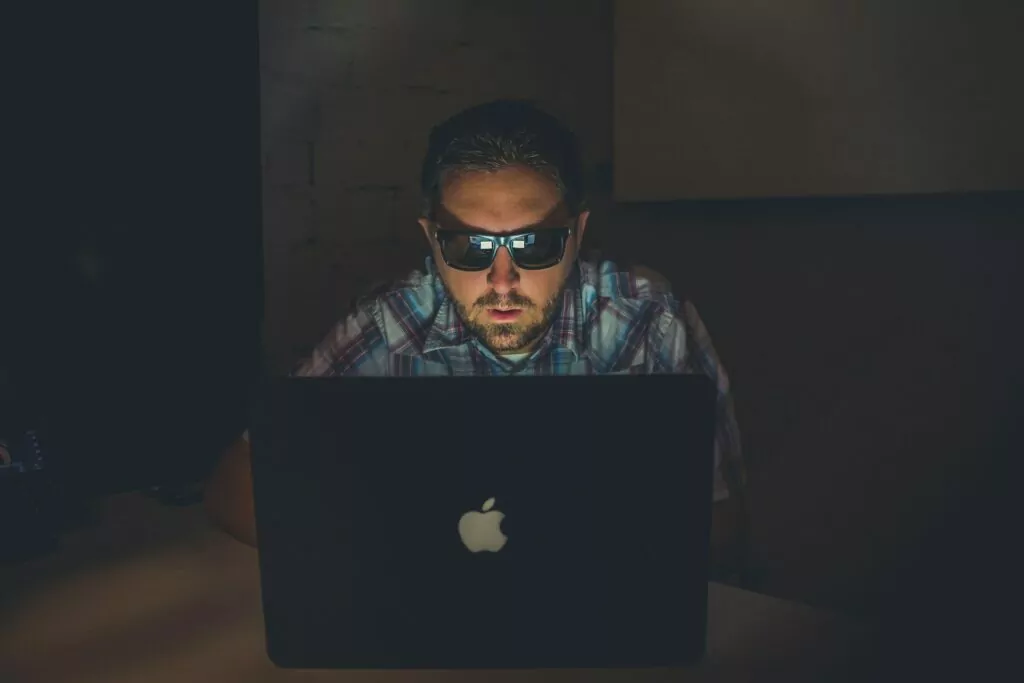
Document Your Reasoning and Assumptions
Transparency in your process is essential — both for collaboration and for bias mitigation. Document how conclusions were reached, including what evidence was used, what was discarded, and why.
Benefits:
- Makes your work more defensible in the event of challenge or scrutiny.
- Helps you revisit past assessments to refine or revise conclusions with new evidence.
- Supports better peer review and organisational learning.
Where possible, annotate your findings with source reliability ratings and confidence levels.
Build in Time for Reflection and Review
Tight deadlines often amplify bias, as there’s little opportunity to question results. Wherever possible, build in time to reflect on findings and review them with fresh eyes.
Tips:
- Schedule a “cooling-off” period before finalising assessments, especially on complex or high-risk topics.
- Use checklists to perform a final bias audit before dissemination.
- Encourage cross-team or external feedback if time allows.
Bias in OSINT is inevitable — but it doesn’t have to define the quality of your work. With the right tools, habits, and organisational culture, it’s possible to create intelligence products that are more balanced, resilient, and actionable.
Embedding Bias Awareness into OSINT Workflows and Culture
Bias mitigation shouldn’t just be left to individual analysts — it must be baked into the wider workflows, processes, and culture of any team or organisation that relies on OSINT. When bias awareness becomes part of the operational fabric, the result is more reliable intelligence, better decision-making, and a stronger ethical foundation.
Here’s how teams can embed this mindset more broadly:
Establish Clear Intelligence Requirements
Start with a well-defined intelligence question. Vague or overly broad tasks increase the risk of confirmation bias or irrelevant collection.
What this looks like:
- Define the “who, what, when, where, and why” before collection begins.
- Break down large requests into smaller, more focused components.
- Ensure tasking is reviewed and agreed by relevant stakeholders to reduce personal bias shaping direction.
Standardise Collection and Documentation Processes
Create workflows that encourage consistency and transparency at every stage of the OSINT cycle.
Steps to implement:
- Use templates for reporting and note-taking that include fields for source evaluation, confidence levels, and assumptions.
- Standardise how tools and sources are chosen and justify their use.
- Make documentation a non-negotiable part of your intelligence output.
This not only reduces bias but also improves reproducibility and quality control.
Foster a Culture of Challenge and Peer Review
Healthy teams encourage respectful disagreement and regular feedback. Challenge should be seen not as confrontation, but as a key part of refining thinking.
How to build this in:
- Hold regular review sessions or “intelligence stand-ups” where analysts discuss findings and alternative views.
- Designate a “red team” or devil’s advocate role for larger projects.
- Encourage cross-functional reviews involving technical, regional, or language specialists where possible.
Psychological safety — where analysts feel comfortable voicing concerns or dissent — is key to making this work.
Provide Ongoing Training and Awareness
Bias awareness isn’t a one-off exercise. Continuous professional development helps teams stay sharp, challenge assumptions, and stay updated with new tools or methods.
Training focus areas:
- Cognitive bias and structured analytic techniques.
- Source validation and reliability frameworks.
- Diversity in online platforms and information ecosystems.
Don’t overlook the value of non-technical skills, such as critical thinking, logic, and media literacy.
Use Technology Thoughtfully, Not Blindly
Automated tools can speed up analysis, but they can also entrench bias if they’re not used carefully. Algorithms are only as objective as the data and assumptions behind them.
Best practices:
- Understand the limitations of any tool, especially those that involve language processing, sentiment analysis, or trend detection.
- Regularly assess whether tools introduce their own selection bias (e.g. geolocation limits, language barriers).
- Avoid over-reliance on dashboards or outputs without context — always layer automated findings with human judgment.
Reflect and Evolve
Build regular retrospectives into your team’s rhythm. Reflect on where bias may have influenced past projects, and use that to refine future practice.
Prompts to consider:
- Were any key perspectives or sources missed?
- Were assumptions tested adequately?
- How did the team handle dissent or uncertainty?
This institutional learning helps embed bias mitigation into your organisational muscle memory.
By putting these cultural and procedural supports in place, organisations move beyond individual effort and towards systemic resilience. When bias awareness becomes a shared value — not just a box-ticking exercise — the result is a more ethical, accurate, and credible OSINT function.
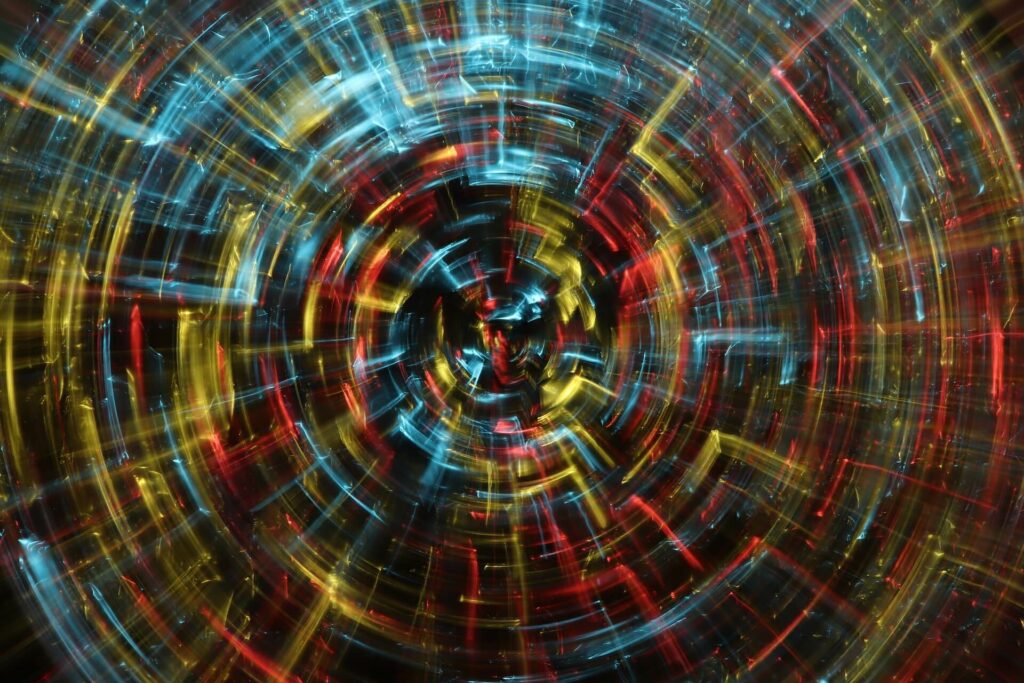
The Value of Bias-Aware OSINT
Bias is an unavoidable part of human thinking, and by extension, of open-source intelligence. But acknowledging its presence isn’t a weakness — it’s a strength. When analysts and organisations recognise where bias can occur and actively work to reduce its influence, the result is not only better intelligence but also more ethical, credible, and impactful work.
Bias-aware OSINT isn’t about striving for some mythical state of total objectivity. Instead, it’s about developing good habits: questioning assumptions, diversifying sources, documenting reasoning, and creating space for challenge and reflection. It’s about embedding checks and balances into both individual workflows and team culture.
In an era where misinformation spreads quickly and decision-makers rely heavily on timely, accurate information, the stakes for getting OSINT right have never been higher. Building bias-aware practices into your investigations isn’t just good tradecraft — it’s an essential part of being a responsible intelligence professional.
By staying curious, critical, and collaborative, we can all do our part to ensure the intelligence we produce stands up to scrutiny and serves its intended purpose — helping others make better-informed decisions.
Header photo by Christian Lue on Unsplash.
Recent Comments