In the evolving landscape of cyber threats, DDoSIA has emerged as a significant force, orchestrating distributed denial-of-service (DDoS) attacks against organisations worldwide. Believed to be operated by pro-Russian hacktivist groups, DDoSIA mobilises volunteer participants to overwhelm targeted networks, causing disruptions to businesses, government institutions, and critical infrastructure. With its decentralised approach and sustained campaigns, this operation has become a persistent threat to cybersecurity resilience.
Tracking DDoSIA is crucial for cybersecurity and threat intelligence (CTI) professionals. By understanding its tactics, techniques, and infrastructure, defenders can better anticipate attacks, mitigate their impact, and adapt defensive strategies. As part of our mission at SOS Intelligence, we continuously monitor, collect, and analyse DDoSIA-related data, offering actionable intelligence to help organisations stay ahead of this evolving threat.
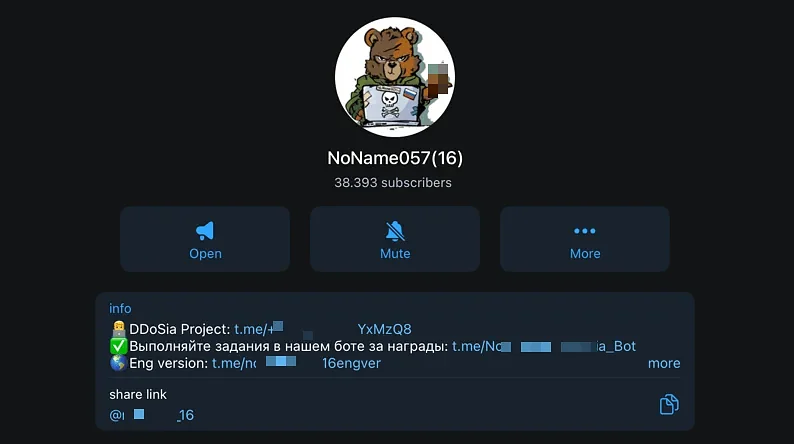
Understanding DDoSIA and Its Attack Infrastructure
DDoSIA is a coordinated distributed denial-of-service (DDoS) campaign operated by pro-Russian hacktivist groups, notably NoName057(16). This group, along with other affiliated threat actors, is known for conducting disruptive cyber operations against organisations and governments deemed hostile to Russian interests. NoName057(16) has been active since at least 2022, launching frequent DDoS attacks against Western institutions, particularly those supporting Ukraine. The group operates as part of a broader ecosystem of pro-Russian cyber collectives, often aligning with entities like KillNet and Anonymous Russia, which share similar geopolitical motivations.
Unlike state-sponsored advanced persistent threats (APTs) that focus on espionage or destructive cyberattacks, DDoSIA is a crowdsourced DDoS initiative, incentivising participants to join attacks. Volunteers—many of whom are ideologically aligned with Russia’s geopolitical stance—are recruited via messaging platforms and forums, where they receive instructions and access to attack tools. Participants are often encouraged through financial rewards or patriotic motivations, making DDoSIA a hybrid between hacktivism and cyber warfare.
How DDoSIA Operates
DDoSIA primarily leverages volumetric and application-layer DDoS attacks, aiming to overwhelm websites, APIs, and network infrastructure. Attack vectors include:
- HTTP flooding – Generating large numbers of HTTP requests to exhaust server resources.
- UDP and TCP floods – Saturating network bandwidth with high-volume traffic.
- Slowloris attacks – Holding connections open to deplete available server connections.
- Bot-assisted attacks – Some participants utilise proxy networks and automated scripts to scale up attack intensity.
The group has targeted various sectors, including government agencies, financial institutions, defence contractors, and logistics providers. A particular focus has been placed on countries actively supporting Ukraine, such as the UK, the US, Poland, and Germany. Attack campaigns often coincide with key political events, military aid announcements, or sanctions imposed against Russia, demonstrating a coordinated cyber-influence strategy.
The Importance of Real-Time Intelligence
Given DDoSIA’s adaptive tactics and decentralised operational model, real-time intelligence is critical for understanding and mitigating its impact. Traditional DDoS mitigation measures alone are insufficient, as the threat landscape evolves rapidly. Continuous monitoring of:
- Attack infrastructure changes (e.g., new command-and-control nodes, shifting IP ranges).
- Recruitment activities in underground forums and messaging platforms.
- Indicators of compromise (IOCs) and attack patterns.
…enables cybersecurity teams to stay ahead of threats.
At SOS Intelligence, we actively track, collect, and analyse DDoSIA-related intelligence, helping organisations anticipate attacks, implement proactive defences, and mitigate operational disruptions before they escalate. By leveraging OSINT, deep web monitoring, and network telemetry, we provide actionable insights to counter the evolving tactics of DDoSIA and its affiliates.
Analysis, Evaluation, and Recommendations
Understanding DDoSIA’s Attack Trends
Unlike financially motivated DDoS campaigns, which often involve extortion or ransom demands, DDoSIA’s attacks are ideologically driven and aim to disrupt services in nations perceived as adversaries of Russia.
Since October 2024, SOS Intelligence has been collecting data from the DDoSIA network, the analysis of which provides critical insight into DDoSIA’s recent campaigns, revealing its geopolitical focus, attack methodologies, and targeted infrastructure. The findings help contextualise the scope of the operation, exposing which nations, industries, and services are most affected.
1. Top Targeted Countries
The distribution of attacks by country reveals a strategic effort to disrupt organisations aligned against Russian interests. The most targeted nations include:
- Ukraine – Consistently the most heavily attacked country, aligning with DDoSIA’s broader mission to destabilise Ukrainian institutions and weaken its digital infrastructure. The targeting of government agencies, financial institutions, and media organisations suggests an attempt to create operational disruption and information blackout scenarios.
- Poland & the Baltic States (Lithuania, Latvia, Estonia) – These nations have been frequent targets of Russian-aligned cyber campaigns due to their strong support for Ukraine. Their strategic position in NATO and the EU’s Eastern flank makes them key adversaries in Russia’s hybrid warfare strategy.
- Western European Nations (France, Germany, UK, Italy, Spain) – The presence of these countries in DDoSIA’s targeting list suggests an attempt to undermine NATO members and critical Western businesses, particularly those providing support to Ukraine.
- Czech Republic & Slovakia – These Central European nations have seen increasing attacks, likely due to their role in military aid and logistical support to Ukraine.
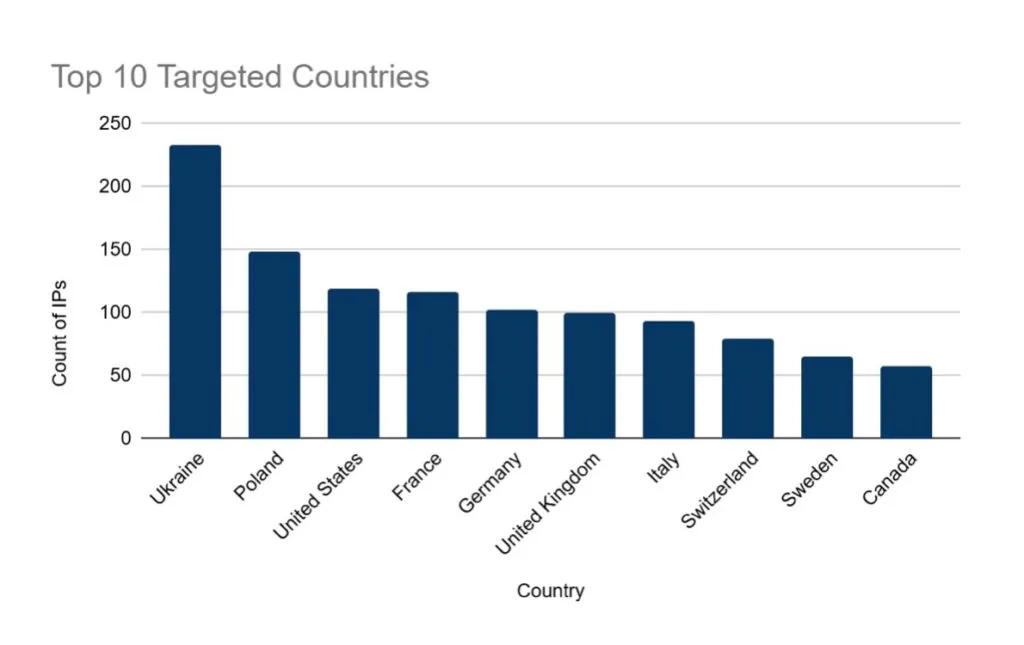
Evaluation
The targeting strategy aligns with broader Russian state-aligned cyber operations, which aim to erode public trust in institutions and disrupt critical services. The focus on government, finance, and media sectors indicates an effort to undermine operational stability and create ripple effects that extend beyond the direct victims.
Implications for Cyber Threat Intelligence (CTI):
- Intelligence gathering on Russian hacktivist groups should prioritise understanding evolving target lists to anticipate future attacks.
- Governments and high-risk organisations in these regions should implement heightened DDoS protections and real-time monitoring to mitigate potential disruptions.
2. Top Victim IPs and Their DDoS Mitigation Status
A key insight from the dataset is the list of IPs that sustained the highest number of DDoS attacks, offering a window into DDoSIA’s strategic intent. The most frequently targeted IPs include:
- Ukrainian Government Infrastructure (91.212.223.216, 18 attacks) – This aligns with previous attacks on Ukrainian state services, attempting to disrupt government communications, digital services, and emergency response systems.
- Microsoft (13.107.246.44 & 13.107.246.61, 14 & 12 attacks) – These IPs are tied to Azure-hosted services, suggesting DDoSIA is attempting to target cloud infrastructure supporting Western businesses or cybersecurity initiatives.
- Polish Banking Networks (193.19.152.74, 10 attacks) – The focus on financial institutions is indicative of an effort to destabilise economic activity in Poland, a strong supporter of Ukraine.
- French E-commerce & Hosting Services (51.91.236.193, 8 attacks) – The targeting of commercial platforms suggests that DDoSIA is testing the impact of attacks on economic stability and supply chains.
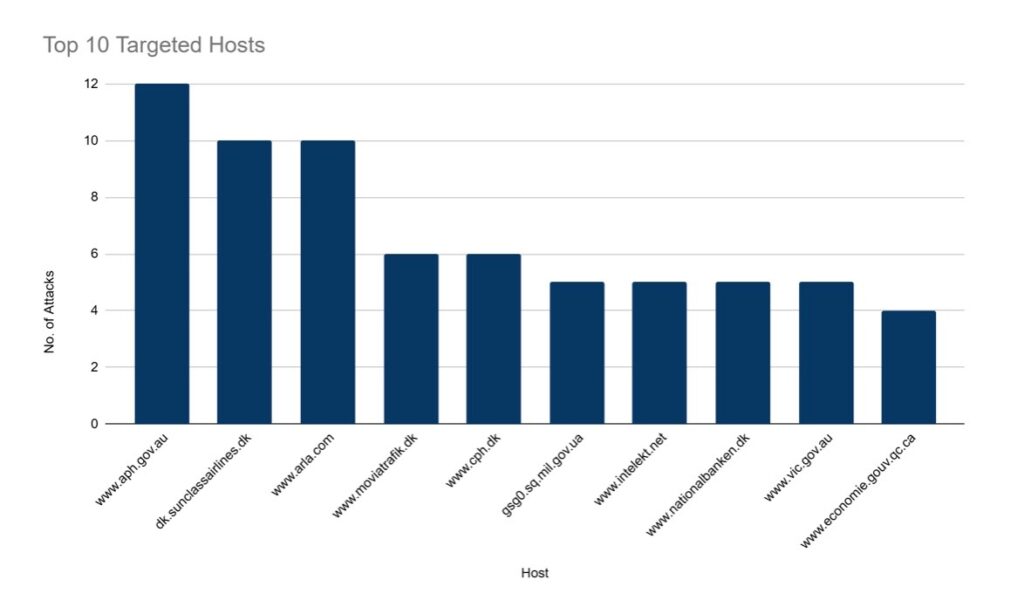
DDoS Mitigation Status Analysis
One of the most notable findings is that many of these victim IPs do not publicly advertise their use of Cloudflare, AWS Shield, or other major DDoS mitigation services. This raises concerns about their ability to withstand sustained attack campaigns.
- High-profile organisations like Microsoft likely have in-house protections, but the presence of their IPs on the list suggests that attackers are attempting to overwhelm cloud-based services.
- Government infrastructure in Ukraine and Poland appears to be a primary target, reinforcing the need for centralised state-sponsored DDoS defences.
- Smaller financial institutions and e-commerce platforms may lack the necessary defences, leaving them vulnerable to outages.
Evaluation
The data suggests that DDoSIA’s attack strategy is not just about volume but also persistence. By continuously targeting specific IPs associated with critical services, they are attempting to cause prolonged service degradation rather than instant takedowns.
Recommendations:
- At-risk organisations should conduct a full audit of their current DDoS protection measures, ensuring they use enterprise-grade filtering solutions.
- Cloud-based services should enhance their rate-limiting policies to mitigate bot-driven HTTP floods.
- Government agencies should coordinate with cybersecurity providers to implement real-time defence measures.
3. Top Attack Methods and Vectors
DDoSIA utilises a combination of attack techniques designed to bypass basic mitigation measures. The most frequently observed attack vectors include:
- TCP SYN Floods – A classic technique used to exhaust connection resources on servers.
- HTTP GET/POST Floods – Targeting application-layer services, often overwhelming login pages, checkout processes, or API endpoints.
- DNS Amplification – Leveraging misconfigured DNS servers to exponentially increase attack traffic.

Evaluation
The presence of HTTP-layer floods indicates an intentional effort to bypass traditional DDoS filtering, which primarily focuses on volumetric mitigation. The attack patterns suggest that DDoSIA’s botnet includes a mix of compromised systems, VPNs, and residential IPs, making mitigation more complex.
Recommendations
For Organisations at Risk
- Implement Layered DDoS Mitigation
- Use a high-quality DDoS mitigation package, such as Cloudflare, AWS Shield, or Akamai for automated volumetric protection.
- Deploy Web Application Firewalls (WAFs) to filter out malicious HTTP traffic.
- Proactive Threat Intelligence & Monitoring
- Implement network anomaly detection tools to identify and block low-volume, high-impact attacks.
- Use geolocation filtering to block or challenge traffic from high-risk regions.
- Strengthen API & Login Security
- Enforce CAPTCHAs and rate-limiting on login and checkout pages.
- Deploy bot management solutions to detect automated DDoS tools.
For CTI Professionals & Security Teams
- Expand DDoSIA Attribution & Tracking
- Monitor NoName057(16)’s recruitment channels to identify new botnet strategies.
- Use honeypots and deception techniques to study attack behaviour in real-time.
- Enhance Threat Intelligence Sharing
- Collaborate with government agencies and private sector security teams to exchange attack data.
- Track botnet infrastructure and preemptively blacklist high-risk traffic sources.
- Develop & Update DDoS Playbooks
- Conduct regular red team exercises to test DDoS resilience.
- Simulate HTTP-layer and multi-vector attacks to identify weaknesses before adversaries exploit them.
Conclusion
The DDoSIA campaign, orchestrated by the NoName057(16) collective, is more than just a disruptive force—it is a tactically coordinated effort aimed at destabilising key institutions in countries opposing Russian geopolitical interests. The data analysed from recent attacks highlights clear patterns in target selection, attack vectors, and mitigation gaps, providing crucial insights into how organisations can defend against such threats.
The attack data reveals a strong geopolitical alignment, with Ukraine, Poland, the Baltic states, and Western European nations being primary targets. The focus on government agencies, financial institutions, and media organisations suggests an intent to erode public confidence, interfere with economic stability, and control narratives in critical regions. Additionally, the fact that Microsoft-hosted services and Polish banking networks have been frequently attacked underlines the strategic importance of both public and private sector entities remaining highly vigilant.
A notable trend is the increasing use of application-layer DDoS techniques (e.g., HTTP floods, DNS amplification, SYN floods), which require more than just volumetric DDoS mitigation. Attackers are leveraging residential proxies, VPN services, and compromised IoT botnets to make their traffic appear legitimate, complicating detection and response efforts.
DDoS as a Smokescreen for Other Cyber Threats
While DDoS attacks are disruptive, they can also serve as a distraction for more insidious cyber activities, such as:
- Network Intrusions & Data Exfiltration – Attackers may launch DDoS attacks to overwhelm security teams, diverting attention while stealing sensitive data or planting backdoors in the organisation’s infrastructure.
- Ransomware Deployment – A coordinated DDoS attack could mask the initial stages of ransomware infections, where threat actors attempt to move laterally through a network before detonating their payloads.
- Supply Chain Compromise – Threat actors may target cloud-based services or third-party providers with DDoS attacks, creating cascading failures that expose vulnerabilities in interconnected systems.
For security teams, this means that DDoS attacks should never be treated in isolation. Organisations must simultaneously monitor network traffic, logs, and user activity for signs of unauthorised access, privilege escalation, or data exfiltration attempts occurring under the cover of a DDoS event.
Strategic Recommendations
To counteract the risks posed by DDoSIA and other hacktivist-driven campaigns, organisations must adopt a multi-layered cybersecurity strategy:
- Advanced DDoS Protection – Deploy Cloudflare, AWS Shield, Akamai, or on-premise DDoS mitigation solutions, with an emphasis on layer 7 (application-level) attack filtering.
- Real-Time Threat Intelligence & Incident Response – Maintain continuous monitoring of attack trends and collaborate with threat intelligence providers to detect emerging tactics early.
- Cross-Channel Security Visibility – Integrate SIEM solutions and Network Detection & Response (NDR) tools to ensure that security teams aren’t solely focused on DDoS traffic, but also on potential concurrent threats.
- Red Teaming & Attack Simulations – Conduct regular stress-testing of infrastructure and simulate multi-pronged attack scenarios to evaluate how well defensive controls hold up under real-world conditions.
- Enhanced Access Controls & Zero Trust – Implement strict user authentication, segmentation of critical systems, and anomaly detection mechanisms to prevent lateral movement during attacks.
Final Thoughts
The DDoSIA campaign exemplifies the increasingly coordinated and persistent nature of cyber threats that blend hacktivism, cybercrime, and geopolitical objectives. As attack techniques evolve, organisations must move beyond reactive defences and adopt proactive, intelligence-driven security strategies.
Crucially, security teams must recognise that DDoS attacks may not be the endgame—they could be a diversion tactic for deeper, more damaging intrusions. By combining DDoS mitigation with network forensics, endpoint monitoring, and proactive intelligence-sharing, organisations can stay ahead of evolving threats and prevent large-scale disruptions before they take hold.
Ultimately, early detection, rapid response, and holistic cybersecurity visibility will determine whether organisations withstand or succumb to these politically motivated cyber assaults.
How SOS Intelligence Empowers You to Analyse and Mitigate DDoSIA Threats
For organisations looking to take a proactive approach to defending against DDoSIA, SOS Intelligence provides raw and processed data that can be leveraged for deeper analysis. Rather than simply offering static reports, our platform enables security teams to interrogate the data in real-time, uncovering trends, patterns, and attack methodologies that can directly inform defence strategies.
Using our threat intelligence feeds, organisations can:
- Correlate Attacker Behaviour – By analysing historical and live attack data, security teams can identify recurring attack patterns, such as preferred attack vectors, geographic focus, and time-based fluctuations in activity.
- Investigate Victimology – By reviewing which organisations, IP ranges, and services are being targeted, defenders can assess their own risk exposure and determine whether their industry, supply chain, or region is in DDoSIA’s crosshairs.
- Detect Emerging Attack Trends – With access to raw network and attack metadata, users can identify new methods being deployed by DDoSIA before they become widespread. This allows for early countermeasure deployment before adversaries refine their techniques.
- Enrich Internal Threat Intelligence – Security teams can cross-reference SOS Intelligence data with their own logs, SIEM alerts, and network telemetry to detect potential early-stage reconnaissance or ongoing infiltration attempts.
- Assess DDoS Mitigation Effectiveness – By tracking which victims have successfully mitigated attacks, teams can gain insight into which defensive solutions (e.g., Cloudflare, AWS Shield, on-premise filtering) have proven most effective.
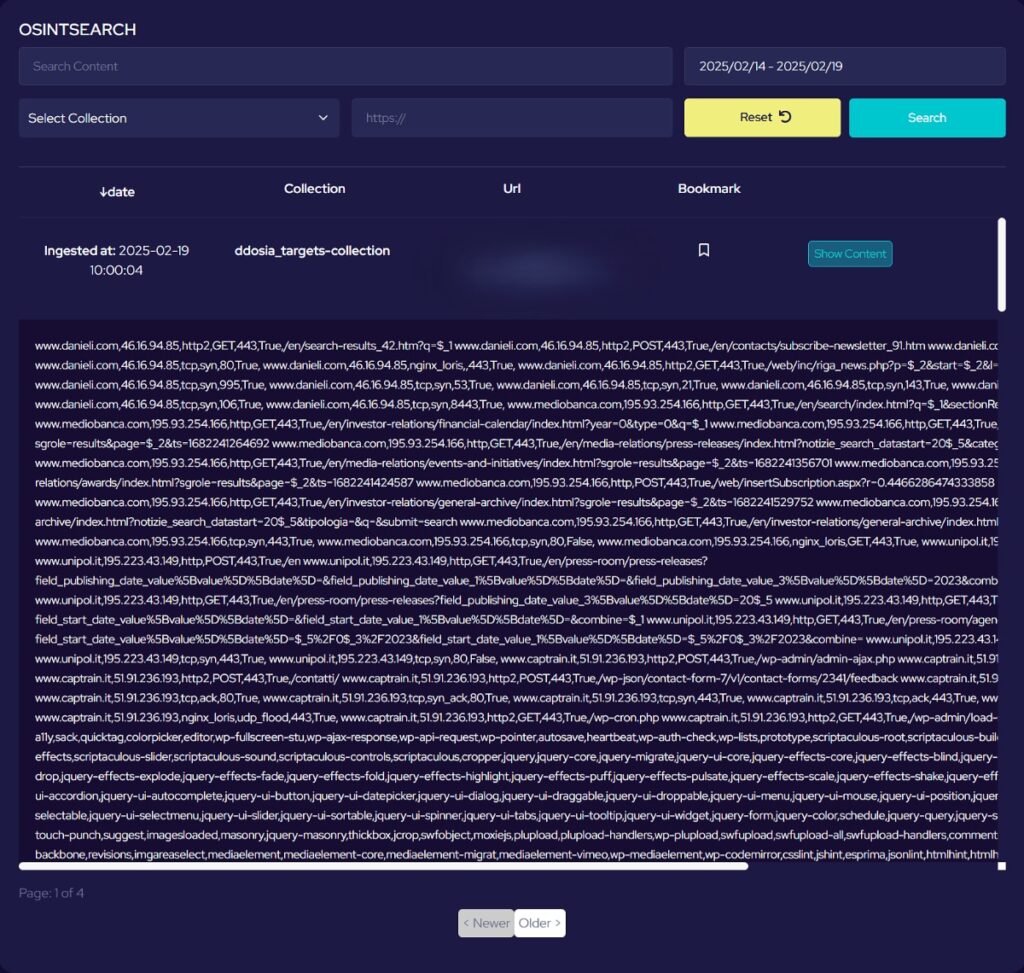
Turning Intelligence into Action
The true value of SOS Intelligence’s DDoSIA data lies in its ability to empower security professionals to extract their own insights. By combining our raw intelligence with in-house security expertise, organisations can:
- Adjust firewall rules and DDoS protection settings based on the latest attack techniques.
- Pre-emptively strengthen defences if they belong to an at-risk industry, country, or sector.
- Monitor attack shifts in real-time to anticipate secondary threats such as network intrusions, data exfiltration, or ransomware campaigns that may accompany a DDoS event.
- Share intelligence within their cybersecurity community to strengthen collective resilience against DDoSIA and similar threats.
Your Intelligence, Your Analysis, Your Defence
SOS Intelligence doesn’t just provide data, it offers a toolset for investigation and insight generation. By leveraging our feeds, logs, and analysis tools, security teams can turn raw data into actionable intelligence, enabling them to detect, understand, and mitigate DDoSIA threats before they escalate.
By combining our intelligence with your expertise, your organisation can stay ahead of DDoSIA’s evolving tactics and transform threat data into a proactive defence strategy.
Header image source – GBHackers.
Recent Comments